We’re moving fast into a world of advanced technology. Here, neuromorphic computing stands out, bringing AI power to processors. It copies how the human brain works, aiming to change how artificial intelligence grows. These new chips might change how we think about processing, creating systems that work more like our brains than traditional computers.
Imagine a chip that works like our brain, learning and fixing mistakes on its own. This is what neuromorphic computing is all about. It’s a big change, not just a small update. It uses less power and can handle changing data better, leading the way in AI tech.
In our search for better tech, neuromorphic computing is a key step. It helps close the gap between today’s AI and the future of brain-like computing. These processors are getting closer to how the brain works, opening up new possibilities for what computers can do.
- Key Takeaways
- Understanding Neuromorphic Computing
- The Evolution of Neuromorphic Chips
- How Neuromorphic Computing Works
- The Significance of Spiking Neural Networks
- Memristors: The Game Changers in Neuromorphic Computing
- Benefits of Neuromorphic Computing
- Real-World Applications of Neuromorphic Computing
- Challenges and Future Horizons
- Conclusion
- External resouces:
- FAQ
- What is neuromorphic computing?
- How does neuromorphic computing differ from traditional computing?
- Who are the key players in neuromorphic computing?
- What role do spiking neural networks play in neuromorphic computing?
- Why are memristors important for neuromorphic computing?
- What are the benefits of neuromorphic computing?
- Can neuromorphic computing be applied in real-world scenarios?
- What challenges does neuromorphic computing face?
- Could neuromorphic computing lead to Artificial General Intelligence (AGI)?
Key Takeaways
- Neuromorphic computing is setting the stage for the next generation of AI-powered processors.
- This advanced technology replicates the function of the human brain, promising enhanced processing power and efficiency.
- Unlike traditional computing models, neuromorphic processors excel in adaptability, consuming far less energy while managing complex tasks.
- A pronounced shift from hardware-focused solutions, neuromorphic chips develop a symbiosis between hardware and software design.
- With an eye on the future, neuromorphic computing is poised to deliver smarter and more agile artificial intelligence systems.
Understanding Neuromorphic Computing
Neuromorphic computing is a new area in computer science. It uses ideas from cognitive computing and brain-inspired computing. These ideas help create systems that work like the human brain. This field is moving away from old computing styles. It could change how we use artificial intelligence (AI) and machine learning.
Defining Neuromorphic Computing
Neuromorphic technology aims to build hardware and software like our brain’s neural networks. These systems do not process info like regular computers. Instead, they work like our neurons and synapses do. This makes AI models work more naturally.
The Brain-Inspired Approach
Neuromorphic systems use neural methods like Spike-Timing-Dependent Plasticity (STDP). This way of learning is based on biology. It lets neuromorphic devices learn and adjust to new info fast. This is similar to how our brains work. Using this idea helps make systems that can handle real-world settings better.
Comparison with Traditional Computing Models
Old computing models have separate areas for storing and processing data. This can slow down data move and use more energy. Neuromorphic computing combines these two parts. This makes information move smoothly, like in our brains. It also makes computers faster and more energy-efficient.
The Evolution of Neuromorphic Chips
Exploring the evolution of neuromorphic chips shows us their impact on artificial intelligence’s future. These chips blend neural processing, semiconductor technology, and complex computer architecture. The shift from ideas to high-tech reality has changed how we compute.
Early Beginnings to Current Advances
The idea of neuromorphic chips began with silicon-based models. These early versions led to today’s advanced designs. Semiconductor technology improved with new materials like memristors. Invented in 1971 and made by HP Labs in 2008, memristors learn like our brains do. They change resistance based on past electric currents. This move to flexible, self-learning processors is a big leap.
Key Players and Technologies
Intel and IBM lead the way in neuromorphic technology. Intel’s Loihi 2 and IBM’s North Pole show big advances. They handle neural tasks better than old chips. Their work pushes the tech forward and sparks more innovation.
Today, we’re at a turning point with neuromorphic chips. They could change how we understand machine learning and AI. As these chips grow more complex, they push us to rethink computational tech’s future.
How Neuromorphic Computing Works
Neuromorphic engineering changes our view of computing as gadgets blend more with human needs. Artificial synapses and neurons copy our brain’s setup. This is a big leap from old computer ways.
Spiking neural networks (SNNs) are key here. Regular networks work non-stop. But SNNs act on special events in time. They process signals by their timing, just like our brains do.
Feature | Traditional Computing | Neuromorphic Computing |
---|---|---|
Processing Method | Continuous and uniform | Spike-based, asynchronous |
Energy Efficiency | Lower due to constant power consumption | Higher, mimics energy-efficient neural processes |
Learning Ability | Static, requires reprogramming for changes | Dynamic, learns and adapts continuously |
Real-time Processing | Limited | Enhanced by intrinsic parallelism and reaction to stimuli |
Spiking neural networks’ special skill is using timing to handle data. They do well with real-time, complex data where old computers can’t keep up.
Neuromorphic engineering is a big step towards smarter AI. It mimics our brain, doing complex tasks well and bringing new AI changes. Making tech more a part of daily life.
The Significance of Spiking Neural Networks
Spiking neural networks (SNNs) stand at the heart of neuromorphic computing. They differ greatly from traditional neural networks used in past AI technologies. SNNs copy the brain’s natural neuron activities. This marks a big step towards machines that process information like humans.
Learning about SNNs helps us see why they’re key for improving neuromorphic hardware. Unlike older networks that always send info, SNNs save energy by activating only when needed. This way, they use less power and do more work, perfect for places with little power.
Understanding Spiking Neural Networks
SNNs process info in a new, dynamic way by using time in their operations. Each neuron fires at specific times, not all the time. This timing helps SNNs act like the brain, leading to machines that learn and react quickly.
Advantages Over Traditional Neural Networks
SNNs have a big edge over old neural networks because they work more like the human mind. They use pulses to work in a non-stop, efficient manner. This and neuromorphic hardware make SNNs faster and better at tasks like recognizing voices and faces.
SNNs don’t just copy the brain’s computing power. They work well with neuromorphic hardware, building a crucial tech for new AI apps. This combination moves us closer to smart systems that do complex tasks using much less power.
Memristors: The Game Changers in Neuromorphic Computing
In the world of neuromorphic computing, memristors are becoming key players. They greatly improve how devices compute and imitate the brain’s architecture. By using these elements, artificial synapses get better. This helps silicon neurons do much more. Let’s explore how these amazing components change technology’s future.
Memristors act as memory elements in an electronic circuit. They remember the electrical charge that has passed through them. This feature lets them hold data even without power, just like our brains save information. It’s similar to how a neuron strengthens from repeated signals.
How Memristors Transform Neuromorphic Computing
Memristors are making a big impact on neuromorphic chips. They represent a huge step away from old computing ways. By doing processing inside the memory, these chips need less data moving back and forth. This cuts down on delay and energy use.
Feature | Traditional Computing | Memristor-based Neuromorphic Computing |
---|---|---|
Data Processing | Sequential and slower | Parallel and faster |
Energy Efficiency | Lower due to frequent data movement | Higher due to localized processing |
Adaptability | Static, fixed programming | Dynamic, learns and adapts |
Size | Larger circuits with separate components | Compact, integrated components |
Putting memristors in neuromorphic chips makes these chips work like the human brain. They become super efficient. As we improve this technology, the future of artificial intelligence could see huge gains.
Read more: Must-Have Software for Remote Job Starters
Read more: “The Future of Robotics: National Strategies and Global Competition
Read more: BioPrinting explanation very simply! 2025
Benefits of Neuromorphic Computing
Neuromorphic computing is a big step forward in technology. It’s known for its great energy efficiency and real-time processing. By adding neuromorphic hardware to our systems, we unlock new and exciting possibilities. This way, we’re getting closer to making machines that work as fast and efficiently as our brains.
This type of computing can manage complex data fast and uses much less power. In a world that cares about saving energy, this is key. Devices with neuromorphic hardware need less help and are perfect for working far away or with the Internet of Things (IoT).
Another big plus is how neuromorphic systems can learn and adapt just like living beings. They’re really good at dealing with the changing world around us. This makes them super useful for a lot of different tasks.
In the end, improving neuromorphic computing will make our technology better, smarter, and more efficient. It guides us towards a future where our technology can react and think more like us.
Real-World Applications of Neuromorphic Computing
In the world of tech, neuromorphic computing is making big changes. It’s boosting how well things work and handling tasks instantly. This new way of computing is very powerful.
Enhancing AI at the Edge
Edge AI is getting a big boost from neuromorphic computing. It makes devices smarter without needing the internet. This is super important for smart cities. It helps them make quick decisions that keep things running smoothly and safely. Neuromorphic chips make things faster and keep data safe, leading to smarter city services.
Revolutionizing Robotics and Autonomous Systems
In robotics and autonomous vehicles, neuromorphic computing is changing the game. Robots and vehicles get neuromorphic processors. This makes them think faster and adapt to changes better. It’s great for industrial robots doing complex tasks on their own. It also helps self-driving cars navigate and stay safe.
Innovations in Healthcare Technology
Healthcare tech is also seeing big benefits. Neuromorphic computing is key for wearable health devices and prosthetics. These can learn about a user’s health and give tailored advice. They help manage daily health and deal with long-term illnesses better.
Neuromorphic computing is really making technology better in our daily lives. It’s making smart cities more efficient and tech in healthcare and cars more responsive. The effects are big and spread far.
Challenges and Future Horizons
As we dive into neuromorphic computing, we find a world full of promise and hurdles. Merging brain-inspired computing and technology is vital for better AI. Yet, this blend is hard to achieve.
Overcoming Technical and Adoption Hurdles
Neuromorphic technology faces technical challenges. Turning complex neural processes into real-world solutions needs teamwork. Scientists and engineers must work together. Also, setting standards for measuring success is crucial to gain investments and acceptance.
There is a push for hybrid systems that mix old and new computing ways. This mix aims to make a powerful system. It would handle tasks like the human brain.
The Road Ahead for Neuromorphic Computing
Neuromorphic computing could hugely change AI across fields. Some think it will help us reach Artificial General Intelligence (AGI). It’s believed to offer unique approaches that enhance today’s AI.
But, we must also think about the ethics and social impact of these technologies. It’s important to develop neuromorphic computing in a fair and ethical way.
- Deepen collaboration between computational neuroscience experts and AI strategists.
- Pioneer robust benchmarks for evaluating neuromorphic systems.
- Enhance public and industrial engagement through transparent and inclusive AI strategies.
We’re at the edge of a tech revolution that could mix human-like processing with computers. This journey is full of both challenges and excitement. Being wise in our approach will be crucial to success.
Conclusion
We’ve explored neurocomputing and its wonders, like neuromorphic architecture and AI-powered processors. We talked about machine learning’s future with these technologies. Neuromorphic computing tries to work like the human brain. This opens up new doors for innovation. Though it has challenges, it offers us a chance to change how AI fits into our lives.
This tech pushes us to rethink information tech and embrace efficient, smart AI processors. For businesses leading in AI, using neuromorphic computing is crucial. It makes our systems not just work, but be smart and adapt. The future of this exciting tech inspires us to create and discover more.
In closing, neurocomputing makes our goals for machine learning and AI clearer. We must face challenges and understand its power to transform. The path forward is as exciting as what we hope to achieve. Every step in neurocomputing promises new advancements we look forward to.
External resouces:
FAQ
What is neuromorphic computing?
Neuromorphic computing copies the human brain to make better computers. It uses special chips to help machines learn and save energy.
How does neuromorphic computing differ from traditional computing?
Unlike traditional computing, neuromorphic computing mixes memory and processing. This mimic brain’s way of working, speeding up data handling and saving power.
Who are the key players in neuromorphic computing?
Intel, IBM, and others lead in neuromorphic computing. They each bring new ideas to make this tech grow.
What role do spiking neural networks play in neuromorphic computing?
Spiking Neural Networks (SNNs) are crucial. They work like brain neurons, making data handling smarter and more efficient.
Why are memristors important for neuromorphic computing?
Memristors are key because they learn like our brains do. They make neuromorphic chips better by being smaller and using less power.
What are the benefits of neuromorphic computing?
Neuromorphic computing is good for tasks that need fast, smart, and energy-efficient processing. It’s great for robotics, smart devices, and more.
Can neuromorphic computing be applied in real-world scenarios?
Yes, it’s used in robots, smart cities, and health gadgets. It helps them make fast, good decisions without needing lots of data or the cloud.
What challenges does neuromorphic computing face?
Challenges include linking brain science to technology, mass production, setting standards, and keeping systems safe from cyber attacks.
Could neuromorphic computing lead to Artificial General Intelligence (AGI)?
Neuromorphic computing might help create AGI due to its brain-like ways. Yet, it’s unclear if it will lead or just help current AI grow.
Get in Touch with SJ Articles
Read more: Screen Time Management Tips That Actually Work
Read more: Remote Ready: Essential Tools for New Work-from-Home Jobs
Read more: Quantum Sensors: Unlocking New Frontiers in Measurement
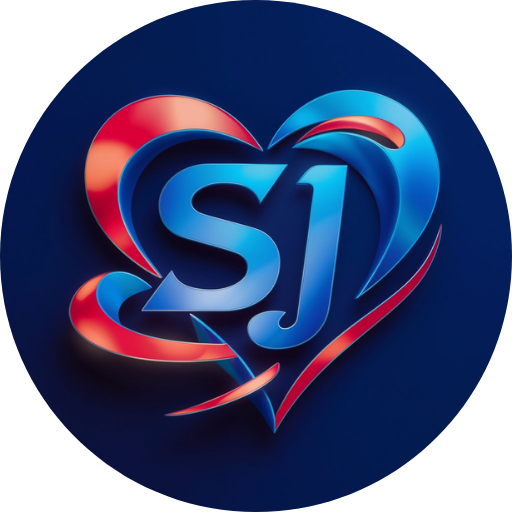