What exactly is multimodal AI?
Before we get to know more about multimodal AI let’s look at its very first name multimodal. In relation to artificial intelligence term “modality” means different types of data as well as modalities. Modalities for data include.. but are not restricted to text audio images & video.
Also multimodal AI can be described as an AI machine.. that has ability to combine and process range of types of input data. inputs to data can include video audio text images as well as various other types of modalities which you’ll discover in next section.
By combining different data types by combining different data types AI system can interpret extensive and diverse array of information. It is soon able provide accurate predictions.. that resemble human beings. In processing these inputs multimodal AI generates an output complex which is contextually conscious.
It is distinct from outputs produced by monomodal systems (as they are dependent upon single type of data).
Multimodal AI examples
Multimodal AI is advancing across diverse fields & is combining kinds of data in order to produce powerful and flexible outputs. few notable examples include:
- GPT-4V(ision)is an upgraded version of GPT-4.. that is able to process texts and images which means.. that AI is able to create visually appealing material.
- The Inworld AI allows for creation of dynamic and interactive characters for games as well as different digital worlds.
- Runway Gen 2 uses text-based prompts to create videos.. that are dynamic.
- DALL-E3DALL-E 3is an OpenAI-based system which produces high-quality images using text-based commands.
- ImageBind from Meta AI uses six different data types such as images text thermal depth and audio for outputs.
- Google’s Multimodal Transformer (MTN) Combines audio text as well as images in order to create subtitles and video descriptions.
Multimodal AI tools
Numerous advanced tools are helping to improve multimodal nature of artificial intelligence.
- Google Gemini allows integration of images text as well as other types of media to make more comprehend & improve material.
- Vertex AI is machine learning platform from Google Cloud. It also processes different types of data & do tasks such as recognition of images analysing videos as well as other.
- CLIP from OpenAI is able to process texts and images in order to complete tasks such as visual search or captioning of images.
- Hugging Face’s Transformers are able to support multimodal learning as well as build flexible AI systems through processing texts audio and pictures.
These systems show.. that multimodal AI is advancing within realm of material making gaming and tackling various other scenarios in real world.
(Know other AIs such as adaptive AI and Generative AI and what is generative AI is for security. )
Multimodal AI is implemented
Multimodal AI operates
AI is an ever-changing area where most recent advancements in algorithms for training to create base models are being applied in multimodal research. field has seen prior advances in multimodality such as audio-visual speech recognition & multimedia material indexing. These innovations was in development before advancements made in deep learning and data science had paved path to next-generation AI.
Nowadays professionals make use of multimodal AI to solve wide range of applications such as analyzing medical pictures for healthcare purposes and together computers for vision in conjunction with other sensory inputs for autonomous vehicles powered by AI.
The 2022 research paper from Carnegie Mellon describes three characteristics of AI.. that are multimodal connectedness heterogeneity as well as interactions.1 Heterogeneityrefers to variety of qualities structure and representations of different modalities. Textual descriptions of particular event could be significantly different in terms of quality structure & presentation in comparison to photo.. that depicts same event.
Connectionsrefers to data.. that is shared among different types of. Connections can manifest by statistical similarity or semantic correspondence. In addition interactions refers to way.. that different types of modalities interrelate when connected.
The main engineering problem of multimodal AI is energetically in integrating and processing different kinds of data into models.. that leverage strengths of every modality as well as overcome limitations of each. In paper authors put following challenges in their paper: representation and alignment reasoning generation transference & quantifying.
points:
- Representationrefers to how you can present and synthesize multiple-modal data so.. that it reflects interdependence and heterogeneity of various modalities. Professionals employ specialized neural networks (for instance CNNs for images transformers for text) to discover features and also employ joint embedding space or attention mechanisms to aid in learning of representations.
- Alignmentaims to determine relationships and interactions between elements. In particular engineers employ methods for temporal alignment of audio and video data as well as spatial alignment in texts and images.
- reasoningaims to synthesize information from multiple sources generally through multiple inferential processes.
- Generationinvolves process of learning for creating modalities in raw form.. that show cross-modal interactions structure & coherence.
- Transferenceaims to transfer information between various modalities. advanced transfer learning methods and sharing embedding spaces enable knowledge to be shared across different various modalities.
Quantification involves a set of theoretical and empirical studies that explore the multimodal nature of learning and evaluate the effectiveness of multimodal models.
Multimodal models add a level of complexity to large language models (LLMs) by utilizing transformers that are based on an encoder-decoder structure, which includes an attention mechanism capable of efficiently handling data. Multimodal AI employs data fusion techniques that combine various types of modalities. The fusion process begins when the model encodes modalities to form an identical representation space, occurs in the middle as different modalities combine at various processing stages, and concludes later when several models process various modalities and mix the results.
The benefits from multimodal AI
There are many advantages to multimodal AI because it has ability to accomplish variety of tasks compared to AI.. that is unimodal. most notable advantages are:
- More contextualization: Multimodal AI analyzes diverse inputs and recognizes patterns. This payoff in authentic and precise outputs.. that resemble human beings.
- Precision: Since multimodal AI blends different streams of data and data sources it could result in better and more precise results.
- enhanced problem-solving: Because multimodal artificial intelligence is able to process variety of inputs it’s able to solve more complicated issues like analysis of media material or determining presence of medical issue.
- Cross-domain learning It allows for efficient transfer of data between multiple modalities which increases ability of data for various purposes.
- Creativity Within realms of material design art and creation of videos Multimodal AI blends data & opening opportunities to develop new outputs.
- Interactive rich: Chatbots Augmented Reality virtual assistants & more make use of multimodal AI to offer additional users with better user experience.
Multimodal challenges AI
Sure multimodal AI has potential to address more problems than systems.. that are unimodal. But as with any other technology at its beginning or developing stage it faces certain issues and disadvantages to it such as ones below.
Higher data requirements
Multimodal AIs will require vast amounts of various information in order to learn definitely. Labeling and collecting these kinds of data can be costly and take long time.
Data fusion
Multiple modalities show various types and levels of noise different times. They do not necessarily have to be in way temporally (time) in sync. Multimodal data renders efficient combination of variety of modalities difficult as well.
Alignment
As with data fusion it’s challenge to coordinate with relevant data in at same time in space with different data types (modalities) are at play.
Translation
Translating material over variety of modalities, for example between distinct modalities or between one language and other is a complicated process referred to Multimodal Translation. Achieving an AI system to produce an image from description of text is an illustration of this type of translation.
Read more: Tech and Mental Health: Striking the Right Balance for Well-Being
Read more: How to Use AI Tools to Save Time Daily
Read more: Antiquantum Encryption in 2025: Essential Facts You Need to Know
One of major challenges in multimodal translation is to make sure.. that model understands meaning of information as well as connections between audio text as well as images. It is also challenging to design models which energetically take in multimodal information.
Representation
The management of various noise levels as well as missing data and need to combine data from different types of modalities are few problems.. that arise with multimodal representation.
Privacy and ethical issues
Like all artificial intelligence technologies there are valid concerns about ethics and security of user.
As AI is developed by humans — individuals with biases bias of AI can be expected. This could result in different outputs based on gender and sexual orientation religious beliefs as well as race and ethnicity.
Additionally AI relies on data for training algorithms. data could contain sensitive personal information. It raises legitimate questions about security concerns regarding Social Security numbers and addresses names and financial details among many more.
(Related read: AI ethical issues privacy of data & AI governance. )
Multimodal AI use cases
Multimodal AI is an exciting advancement.. but it has some way to go. possibilities are almost limitless. ways.. that we could make use of multimodal AI include:
- Enhancing efficiency of autonomous vehicles by combining information from several sensors (e.g. cameras radars and lidar).
- Making new medical diagnostic instruments which make use of information from images scans medical records as well as genetic test outcome.
- Enhancing chatbots and virtual assistant’s experience through processing a range of inputs as well as making more complex outputs. ( Meta has an entertaining prompt to choose if you’d like to give it go.)
- Implementingenhanced protection against fraud and risk assessments in banking financial finance and other sectors.
- An analysis of data from social media comprising images text & videos to enhance material moderation and detection of trends.
- Making robots comprehend and communicate with their surroundings which leads to greater human-like behaviors and capabilities.
With difficulties of carrying out these complicated tasks & ethical and legitimate privacy issues raised by experts it will likely take considerable amount of time until multimodal AI technologies are integrated into our everyday life.
Risks of Multimodal AI
Like any other new technology there are number of possible pitfalls we need to avoid when working with AI models.. that are multimodal:
Insufficient transparency.
Algorithmic transparency ranks among the most significant issues associated with generative AI, and this also holds true for multimodal AI. Experts usually refer to these types of AI as black box models due to their complex nature, which makes it difficult to track their logic and internal workings.
Multimodal AI monopoly.
Due to the huge amount of resources needed to create train and run an AI model.. that is multimodal this market is concentrated within a handful of Big Tech companies with required expertise and resources. There is steady increase of open-source LLMsare entering market and helping developers AI researchers & society to grasp and utilize LLMs.
Discrimination and bias.
Depending on information used in training of multimodal AI models they could have biases.. that could lead to unfair decisions which tend to improve discrimination especially for minority groups. In past importance of transparency for better understanding and addressing the possibility of bias.
Privacy concerns.
Multimodal AI models have been trained on vast quantities of data derived from variety of formats and sources. It is often case.. that it could contain personal information. It can cause issues and threats to security and privacy of data.
Considerations regarding ethics.
Multimodal AI can sometimes lead to decisions with serious consequences for our daily lives which can have significant impact on fundamental rights we enjoy. We examined morality of generative AI in an extra post.
Environmental concerns.
Researchers and environmental monitoring organizations are concerned about the impact of running and training generative AI models on the environment. Owners of multimodal proprietary AI models seldom release data about the amount of energy and other resources used in the models, as well as the environmental footprint. This transparency can be extremely challenging given the rapid growth of these technologies.
Challenges of Implementing Multimodal AI Solutions
The multi-modal AI boom has unlimited possibilities for companies as well as individuals, government agencies and businesses. But like any new technology with them within your day-to-day operations may be challenge.
First it is important to determine accurate use cases to meet your particular requirements. transition from idea to application can be challenge and especially when you are not individuals who understand technical aspects of multimodal AI. In the context of a skills deficit in the market, companies often find it costly and difficult to locate individuals who can put models into production, as they are willing to spend significant amounts of money to acquire this kind of skilled personnel.
When discussing generative AI mention of affordability is must. models particularly multimodal ones demand significant amount of computational resources in order to function which means.. that they will cost you money. So prior to adopting any type of generative AI method you need to calculate budget.. that you’d like to allocate.
The various paths to multimodal AI
In this article, we have shown that the multimodal nature of AI is an essential advancement within AI systems. As researchers conduct more studies, this new technology could enhance AI’s capabilities and transform areas like autonomous driving technologies, healthcare, and many more.
Despite promising times ahead of multimodal AI has its own problems like biases and ethical issues in relation to privacy and amount of data required.
With technology constantly changing as it does we must deal effectively with challenges so.. that we can unlock potential of multimodal artificial Intelligence. While it could take some time for it to collect traction and develop it is likely to improve when it comes to solving problems.. that are complex with humanlike approach within various fields.
Get in Touch with SJ Articles
Read more: Tech and Mental Health: Striking the Right Balance for Well-Being
Read more: How to Use AI Tools to Save Time Daily
Read more: Antiquantum Encryption in 2025: Essential Facts You Need to Know
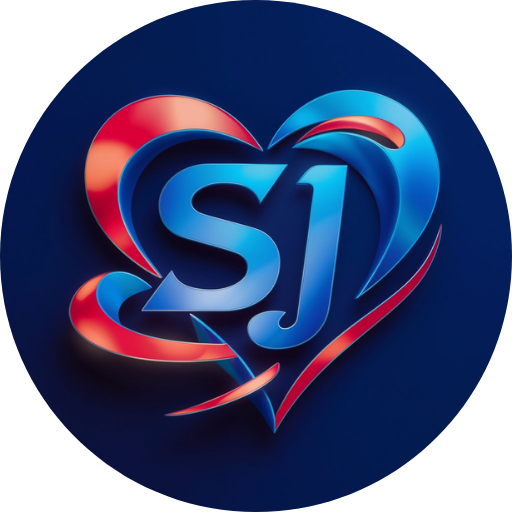